AI for everyone - How companies can benefit from the advance of machine learning
This article titled “Wie Unternehmen vom Vormarsch des maschinellen Lernens profitieren können” appeared in German last week in the “Digitaliserung” column of Wirtschaftwoche.
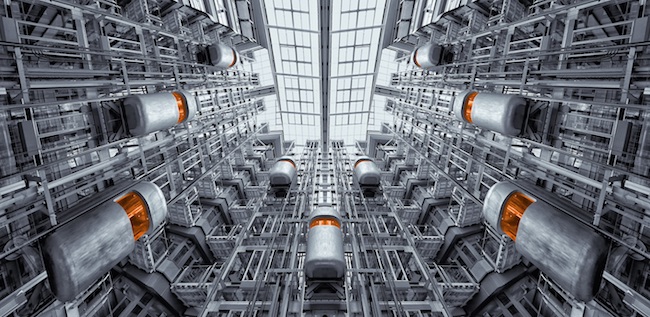
When a technology has its breakthrough, can often only be determined in hindsight. In the case of artificial intelligence (AI) and machine learning (ML), this is different. ML is that part of AI that describes rules and recognizes patterns from large amounts of data in order to predict future data. Both concepts are virtually omnipresent and at the top of most buzzword rankings.
Personally, I think – and this is clearly linked to the rise of AI and ML – that there has never been a better time than today to develop smart applications and use them. Why? Because three things are coming together. First: Users across the globe are capturing data digitally, whether this is in the physical world through sensors or GPS, or online through click stream data. As a result, there is a critical mass of data available. Secondly, there is enough affordable computing capacity in the cloud for companies and organizations, no matter what their size, to use intelligent applications. And thirdly, an "algorithmic revolution" has taken place, meaning it is now possible to train trillions of algorithms simultaneously, making the whole machine learning process much faster. This has allowed for more research, which has resulted in reaching the "critical mass" in knowledge that is needed to kick off an exponential growth in the development of new algorithms and architectures.
We may have come a relatively long way with AI, but the progress came quietly. After all, during the last 50 years, AI and ML were fields that had only been accessible to an exclusive circle of researchers and scientists. That is now changing, as packages of AI and ML services, frameworks and tools are today available to all sorts of companies and organizations, including those that don't have dedicated research groups in this field. The management consultants at McKinsey expect that the global market for AI-based services, software and hardware will grow annually by 15-25% and reach a volume of around USD 130 billion in 2025. A number of start-ups are using AI algorithms for all things imaginable – searching for tumors in medical images, helping people learn foreign languages, or automating claims handling at insurance companies. At the same time, entirely new categories of applications are being created whereby a natural conversation between man and machine is taking center-stage.
**Progress through machine learning **
Is the hype surrounding AI and ML even justified? Definitely, because they offer business and society fascinating possibilities. With the help of digitization and high-performance computers, we are able to replicate human intelligence in some areas, such as computer vision, and even surpass the intelligence of humans. We are creating very diverse algorithms for a wide range of application areas and turning these individual pieces into services so that ML is available for everyone. Packaged into applications and business models, ML can make our life more pleasant or safer. Take autonomous driving: 90% of car accidents in the US can be traced to "human failure". The assumption is that the number of accidents will decline over the long term if vehicles drive autonomously. In aviation, this has already been reality for a long time.
MIT pioneers Erik Brynjolfsson and Andrew McAfee predict that the macroeconomic effect of the so-called "second machine age" will be comparable to what the steam engine once unleashed when it replaced humans' muscular strength ("the first machine age"). Many are uncomfortable with the idea that an artificial intelligence exists alongside human intelligence. That is understandable. We must therefore discuss – parallel to the technological developments – how humans and AI can co-exist in the future; the moral and ethical aspects that arise; how to ensure we have a good grip on AI; and which legal parameters we need in order to manage all this. Answering these questions will be just as important as the effort to solve the technological challenges, and neither dogmas nor ideologies will help. Instead, what's needed is an objective, broad-based debate that takes into account the wellbeing of society as a whole.
Machine Leaning at Amazon
For the past 20 years, thousands of software engineers at Amazon have been working on ML. We dare to claim that we are the company that has been applying AI and ML as a business technology the longest. We know that innovative technologies always take off whenever barriers to entry fall for market participants.
That is the case right now with AI and ML. In the past, anyone who wanted to use AI for himself had to start from scratch: develop algorithms and feed them with enormous amounts of data – even if he later needed an application for a strictly confined context. This is referred to as so-called "weak" AI. Many of the consumer interfaces that everyone is familiar with today, such as recommendations, similarities or autofill functions for search prediction – they are all ML driven. In the meantime, they can predict inventory levels or vendor lead times, detect customer problems and automatically deduct how to solve them; and discover counterfeit goods and sort out abusive reviews, thereby protecting our customers from fraud. But that is only the tip of the iceberg. At Amazon, we are sitting on billions of historical order information data, which allows us to create other AI/ML-based models based on AI for many different kinds of functionalities. For example programming interfaces that developers can use to analyze images, change text into true-to-life language or create chatbots. But ultimately, there is something to be found for everyone who wants to define models, train them, and then scale. Pre-configured, attuned libraries and deep learning frameworks are widely available, which allow anyone to get started very fast.
Companies like Netflix, Nvidia, or Pinterest use our capabilities in ML and deep learning. More and more layers are being created in a kind of ecosystem on which companies and organizations can 'dock' their business – depending on how deep they want to, and are able to, immerse themselves in the subject matter. Decisive is the openness of the layers and the reliable availability of the infrastructure. In the past, AI technologies were so expensive that it was hardly worth it to use them. Today, AI and ML technologies are available off the shelf, and they can be called up according to one's individual requirements. They form the basis for new business models. Even users who are not AI specialists can very easily and affordably incorporate the building blocks into their own services. In particular small and medium-sized companies with innovative strength can benefit. They do not have to learn any complex ML algorithms and technologies, and they can experiment without incurring high costs.
Artificial intelligence helps to satisfy the customer
One of the most advanced areas of application is e-commerce. AI-supported pre-selection mechanisms help companies to free their customers' decision making from complexity. The ultimate goal is customer satisfaction. If there are only three types of toothpaste, the customer can easily pick one and feel good about it. When more than 50 kinds are on offer, the choice becomes complicated. You have to decide, but you're not sure if the decision is the right one. The more possibilities there are, the more difficult it becomes for the customer. Our best-known algorithms come from this field: filtering product suggestions based on one's purchase history of products with similar attributes, or on the behavior of other customers who were interested in similar things.
Of course, consistent quality also contributes to the satisfaction of the customer. Intelligent support makes life easier for the provider and the customer. For Amazon Fresh, for example, we have developed algorithms that learn how fresh groceries have to look, how long this state lasts, and when food should no longer be sold. Airlines or rail transport companies could also use this for their quality control by running an algorithm based on the image data of the freight; the algorithm would recognize damaged goods and automatically sort them out.
If you can predict demand, you can plan more efficiently
In B2B and B2C businesses, it is critical that goods are available quickly. It is for this reason that we at Amazon have developed algorithms that can predict the daily demand of goods. This is particularly complex for fashion goods, which are always available in many different sizes and variations and for which reorder possibilities are very limited. Information about past demand, among others, is fed into our system, as well as fluctuations that can occur with seasonal goods, the effect of special offers, and the sensitivity of customers to price shifts. Today we can predict precisely how many shirts in a certain size and color will be sold on a defined day. We have tackled this issue and made the technology available to other companies as a web service. MyTaxi, for example, benefits from our ML-based service to plan at what time and at which place the customer will need the vehicle.
New division of labor
But AI is much more than just forecasting. In the field of fulfillment, which is relevant for numerous industry sectors, we are thinking of ideas of how AI can contribute the most to taking another step away from a Tayloristic work pattern. Applied in robots, AI can free people from routine activities that are physically difficult and often stressful. Machines are very good at, and sometimes even outperform, tasks that are complicated for a human to do, such as finding the optimal route in a warehouse for a certain number of orders and transporting heavy goods to the point where it is sent to the customer. For supposedly easy tasks, by contrast, the robot is overwhelmed; an example is recognizing a box that has landed on the wrong shelf. So how to bring together the best of both players? By letting intelligent robots learn from humans how to identify the right goods, take on various orders and navigate their way autonomously through the warehouse on the most efficient route. This is how we take away the most tedious part of the task and shift resources to more interaction with the customer.
Our client SCDM uses the core idea of freeing up resources for "human" strengths, but in a completely different context. SCDM is a service provider that supports banks and insurance companies with digitization. Using AI, SCDM enables its customers to classify documents that are of very different formats (PDF, Excel or photos), for example a report about the performance of an investment product that contains hundreds of pages. By scanning hundreds of thousands of documents simultaneously, SCDM's algorithm recognizes which document is relevant for a specific request, finds out where relevant data for a specific type of preparation is located, and then extracts the data from the document. As a result, there is less bias and fewer errors in the number crunching, and more time for human interaction with important stakeholders like investors, analysts and other customers.
Machine learning in education, medicine and development aid
In addition to their potential for things like efficiency and productivity, ML and AI can also be used in education. Duolingo, which offers free language course apps, uses text-to-language algorithms to assess and correct learners' pronunciation. In medicine, AI supports doctors in analyzing X-Ray CTs or MRT images. The World Bank also uses AI in order to implement infrastructure programs, development aid and other measures in a more targeted manner in the future.
More room for optimism
Despite all these developments, many people from academia, business and government have a critical view of ML and AI. There have been warnings that a new super-intelligence is jeopardizing our civilization – and these warnings have been effective in attracting publicity.
However, neither hysteria nor euphoria should be allowed to get the upper hand in the public debate. What we need instead is a pragmatic-optimistic view of the emerging possibilities. AI enables us to get rid of tasks in our work which damage our health or where machines are better than we are. Not with the goal of making ourselves redundant. Rather, in order to gain more personal and economic freedom – for interpersonal relationships, for our creativity and for everything that we humans can do better than machines. That is what we should strive for. If we don't, we will ultimately forego the economic and societal opportunities that we could have grasped.